AI TotalOps : Data, ML & Security
What is AI TotalOps : Data, ML & Security and how do they work together?
At NSigma, our AI TotalOps: Data, ML & Security offering represents the pinnacle of operational excellence tailored for the evolving landscape of Data Science and AI/ML innovations. AI TotalOps is built on a foundation of four critical pillars—DataOps, MLOps, DataSecOps, and AIOps—each infused with the principles of continuous integration and continuous delivery (CI/CD) from DevOps. This cohesive framework empowers organizations to innovate rapidly while maintaining high standards of data integrity, model reliability, and system security.
What Constitutes AI TotalOps : Data, ML & Security
DataOps
DataOps emphasizes a streamlined, automated approach to managing data workflows, from acquisition to insight. It integrates practices from software development and data science to enhance the quality, speed, and governance of data analytics. By fostering collaboration across data managers, engineers, and analysts, DataOps ensures that data is not only accurate and accessible but also delivered in real-time to stakeholders, enabling more agile and informed decision-making
Learn MoreMLOps
MLOps combines machine learning, data science, and operations to streamline the lifecycle of ML models from development to deployment and maintenance. This methodology ensures that ML models are scalable, reproducible, and maintainable, facilitating seamless collaboration between data scientists and operations teams. MLOps aims to accelerate the transition of models from experimentation to production, optimizing their performance and ensuring they continue to deliver value over tim
Learn MoreDataSecOps
DataSecOps integrates security principles into the data management and analytics lifecycle, ensuring that data privacy, compliance, and protection are maintained throughout the process. By embedding security measures from the onset of data projects, DataSecOps proactively addresses potential vulnerabilities, safeguarding against data breaches and leaks. This approach ensures that data not only drives insights but does so in a manner that respects and protects sensitive information.
Learn MoreAIOps
AIOps applies artificial intelligence to IT operations, leveraging machine learning and analytics to automate and enhance IT processes. It focuses on analyzing big data collected from various IT operations tools and devices, to predict and prevent issues in real time, optimize performance, and support decision-making. AIOps enables organizations to manage the complexity and volume of data in their IT environments more effectively, leading to more resilient and responsive IT services.
Learn MoreAI TotalOps: Data, ML & Security
The synergy and workflow integration among these components can be understood in three main stages:
1) DataOps Adopts DevOps Principles
DataOps leverages the agility and efficiency of DevOps, particularly CI/CD, to enhance data management practices. This method ensures seamless data integration, quality, and availability, providing a robust foundation for analytics and machine learning initiatives. DataOps serves as the cornerstone, prioritizing the flow of clean, reliable data across the organization, thus enabling informed decision-making and rapid insight generation
2) MLOps Extends Beyond DataOps
Emerging from the advanced practices of DataOps, MLOps focuses on overseeing the complete lifecycle of machine learning models. This includes development, training, evaluation, deployment, and ongoing monitoring, all underpinned by CI/CD methodologies. MLOps and DataOps are intrinsically linked; as DataOps guarantees a continuous supply of quality data, MLOps leverages this asset for building and maintaining models that are precisely tuned to the organization’s objectives. The dynamic between MLOps and DataOps is characterized by their interdependence, fostering an environment where data feeding and model optimization occur in a concurrent, streamlined fashion.
3) DataSecOps Embeds Security within DataOps and MLOps
Replacing DevSecOps, DataSecOps integrates security practices directly into the DataOps and MLOps workflows. This approach ensures that from the earliest stages of data handling and model training, through to deployment and application, every process is secured and compliant with relevant standards. DataSecOps not only protects against data breaches and leaks but also secures the machine learning models from potential vulnerabilities, making security a continuous aspect of the operational process.
4) AIOps Enhances TotalOps with AI-Driven Insights
AIOps stands as the fourth pillar within AI TotalOps, utilizing AI to automate and optimize IT operations. It brings predictive capabilities, anomaly detection, and real-time insights into system performance and security threats, further enhancing the efficiency and effectiveness of DataOps, MLOps, and DataSecOps. AIOps acts as the neural network of TotalOps, intelligently connecting and optimizing operations across the board.
NSigma AI TotalOps: Orchestrating Operational Harmony
AI TotalOps: Data, ML, and Security is more than a framework; it's an orchestrated methodology harmonizing CI/CD principles with the specialized needs of data management, machine learning, and security. It ensures that NSigma’s clients stay ahead in the fast-evolving AI landscape, innovating securely and sustainably at the speed of thought.
Signs your business could use AI TotalOps : Data, ML & Security
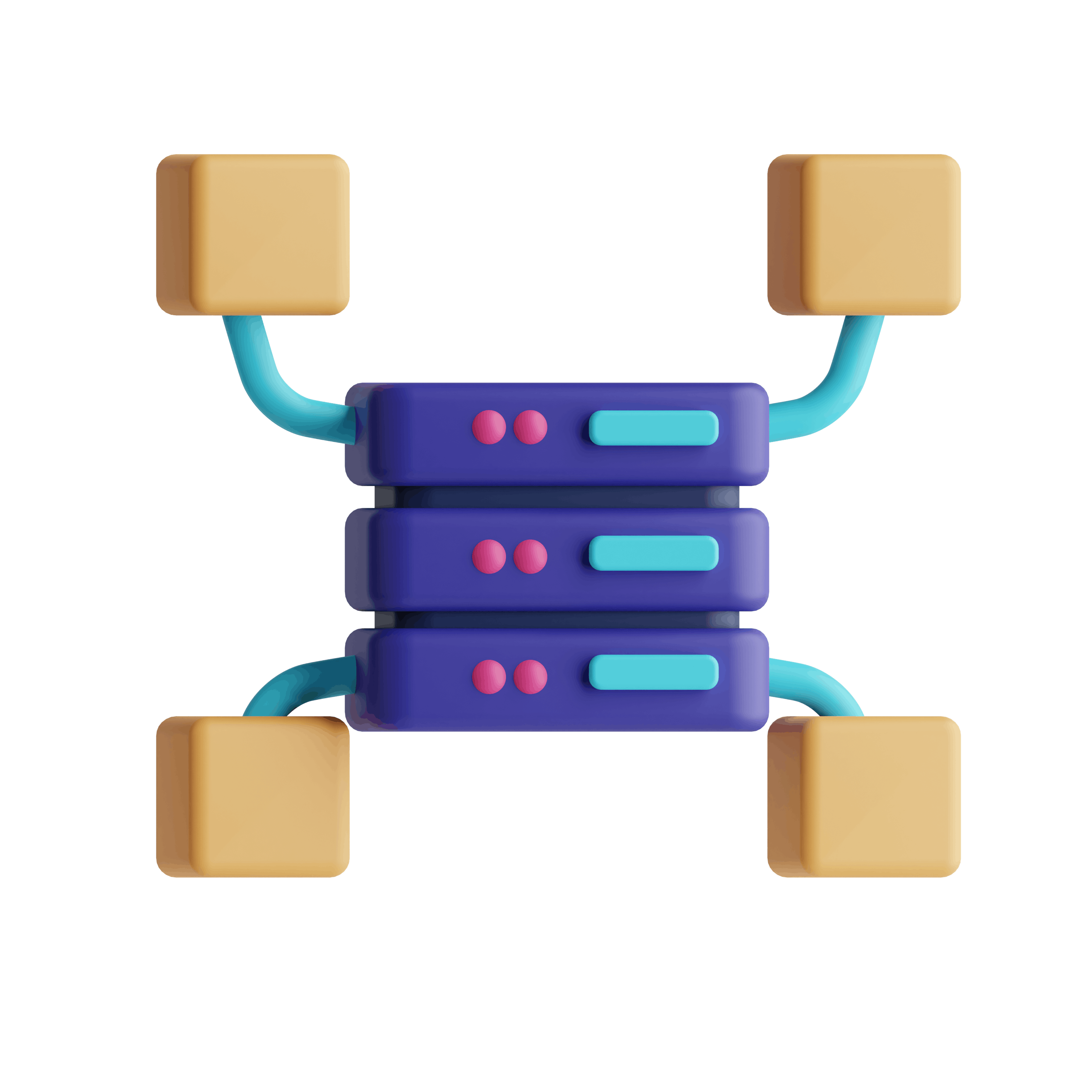
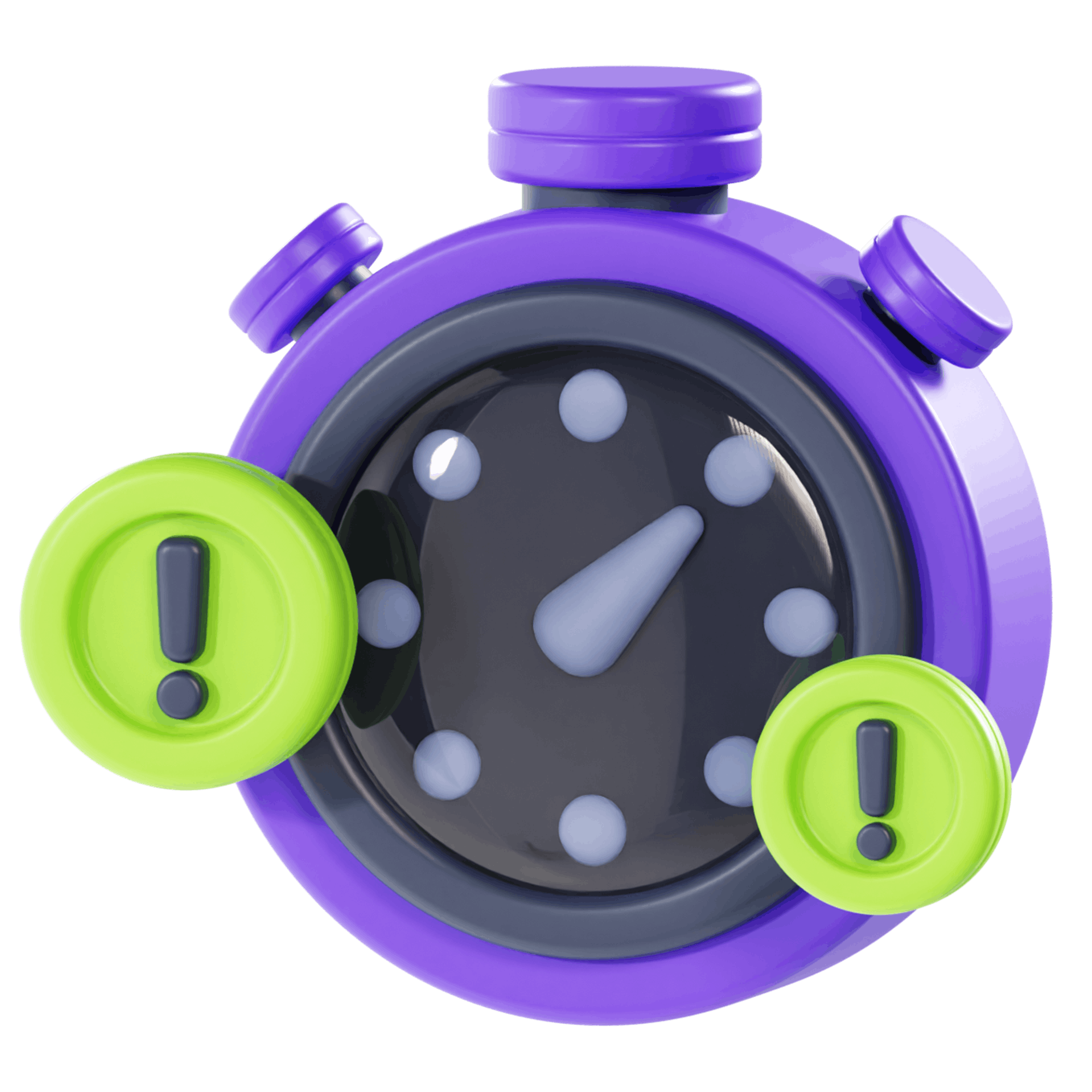
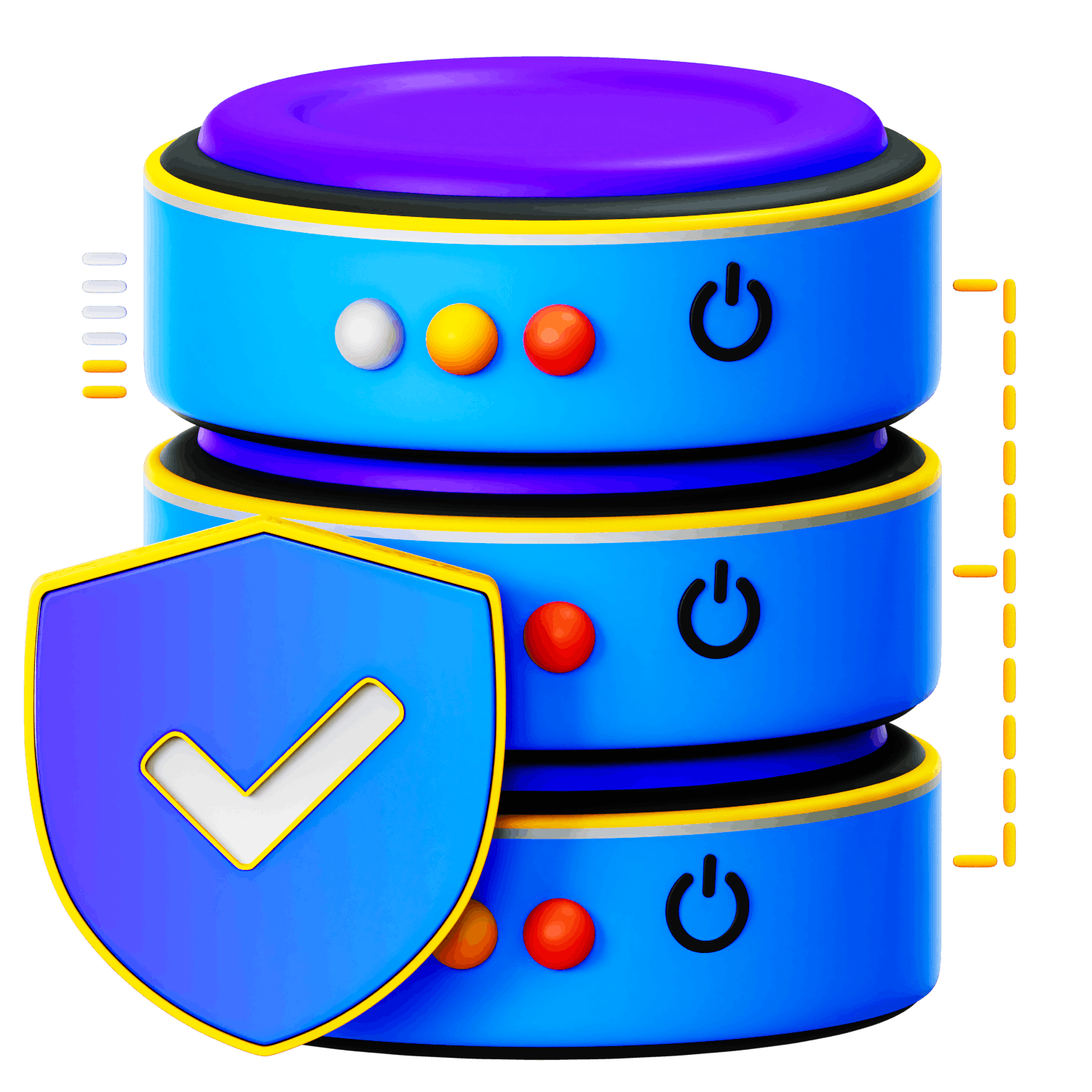
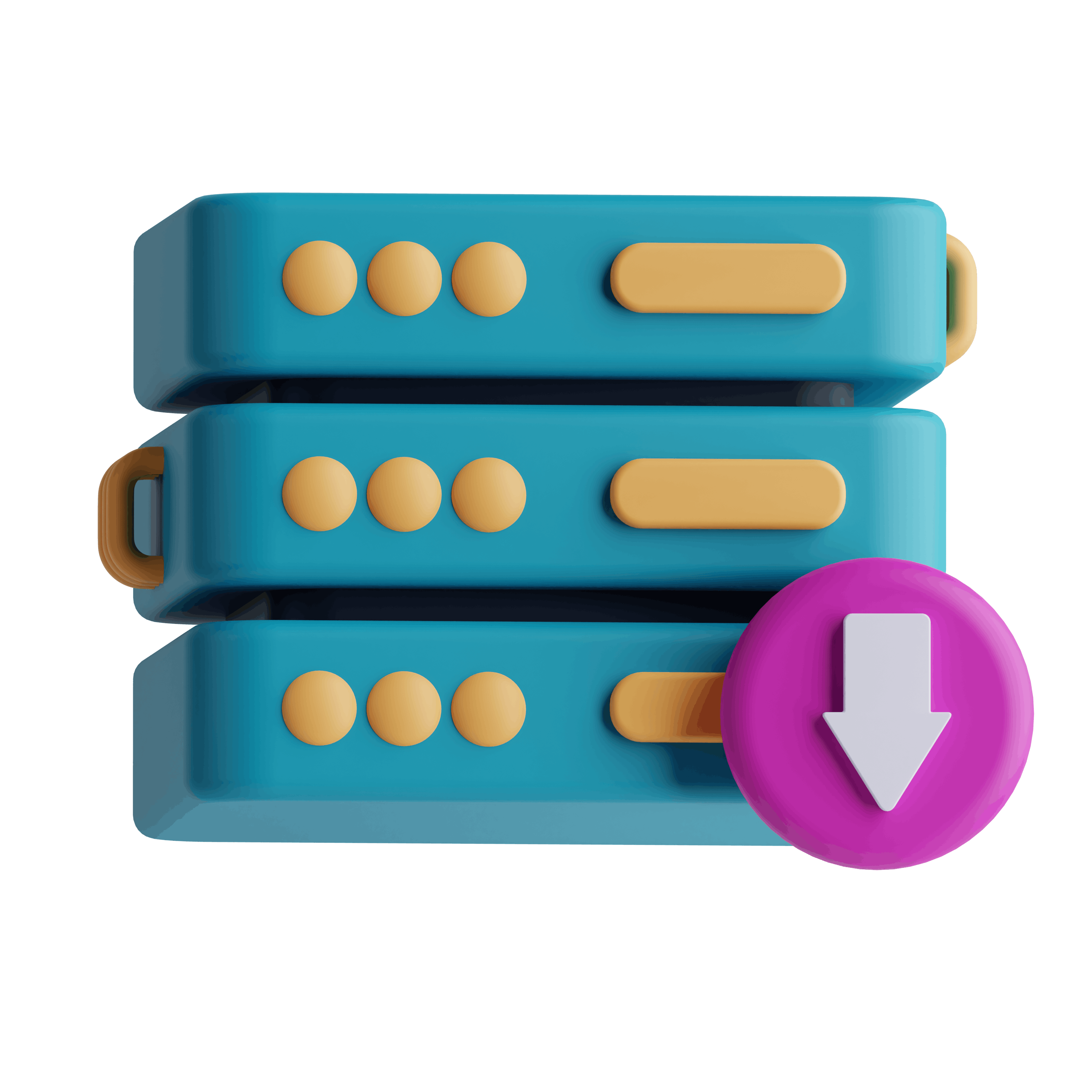
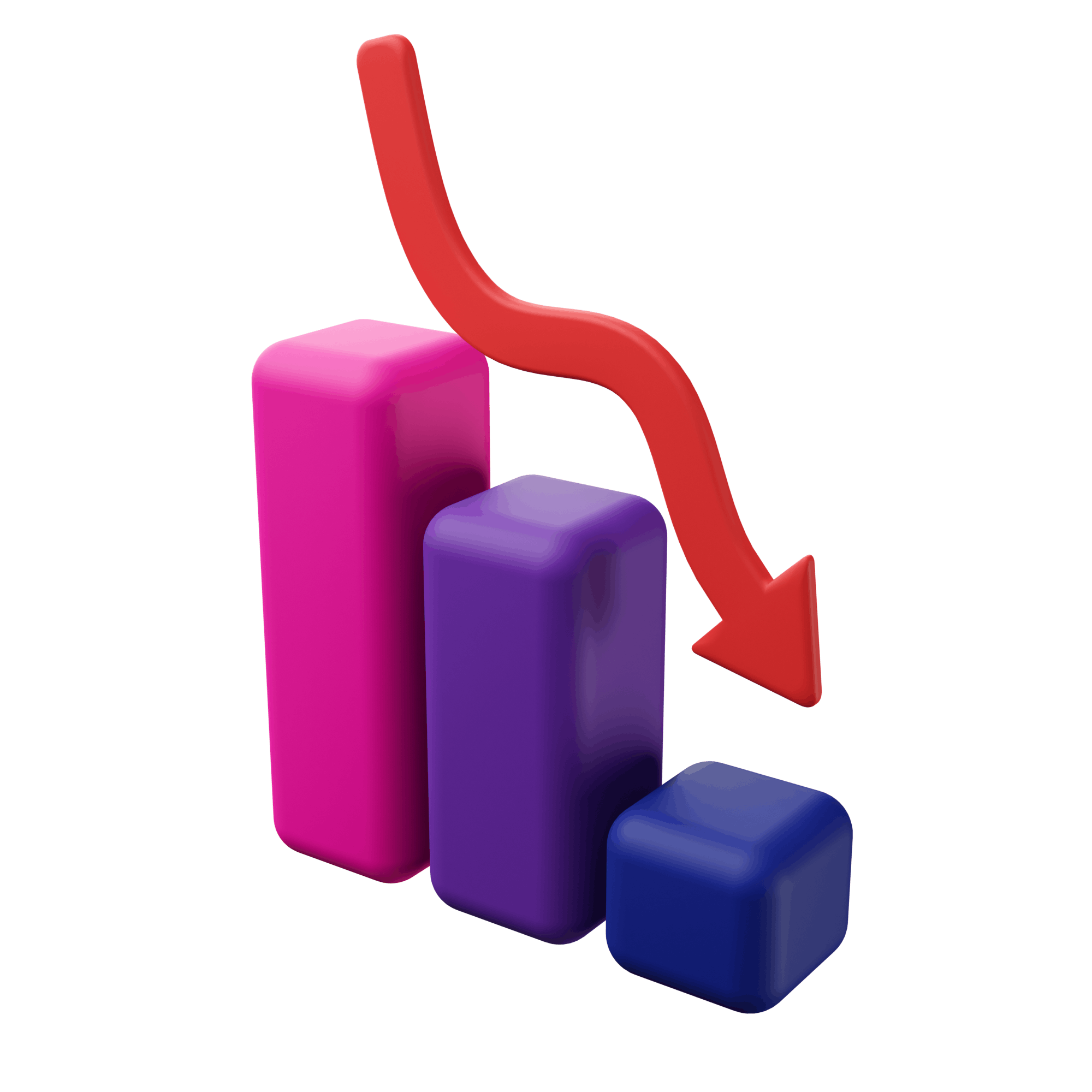
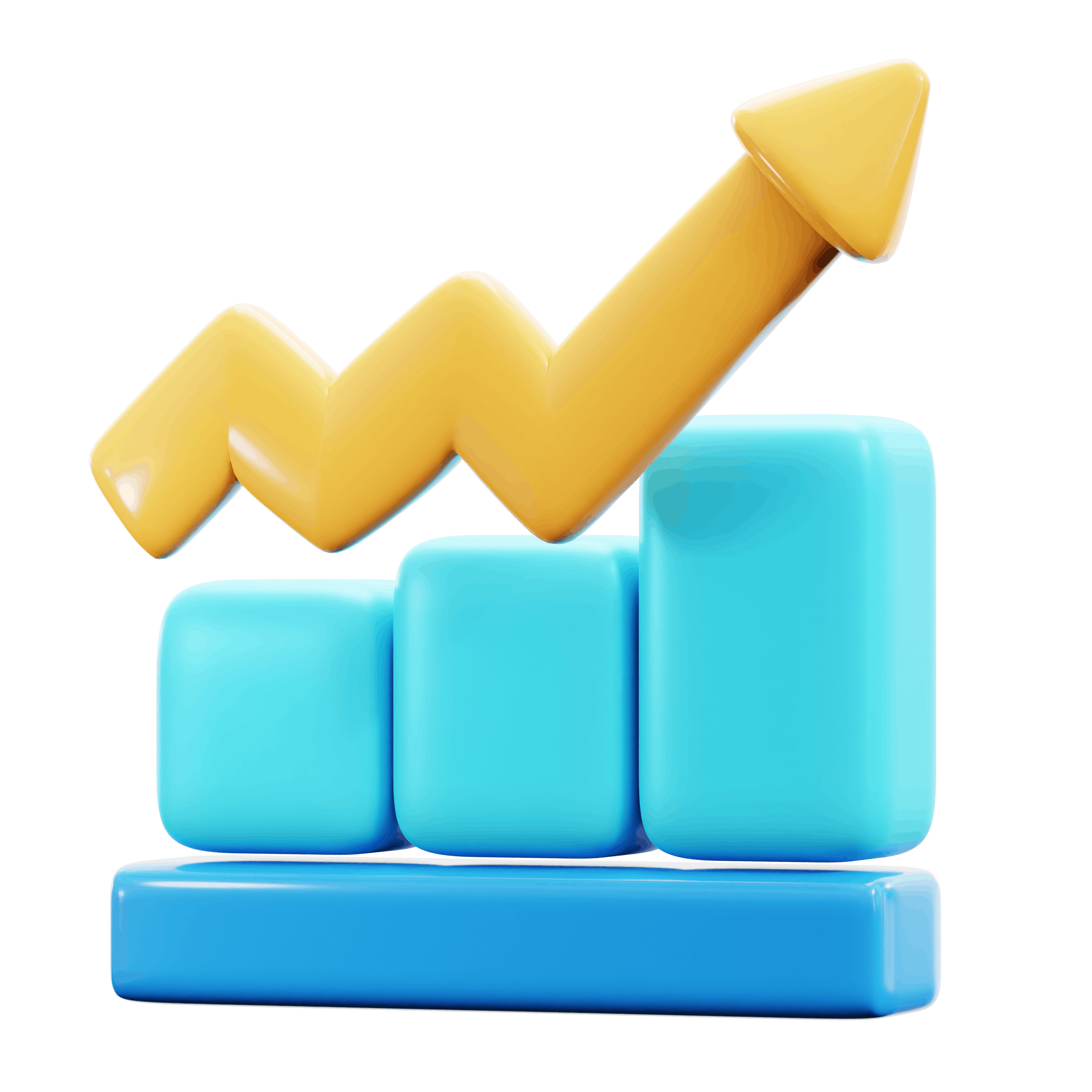
Benefits of AI TotalOps : Data, ML & Security
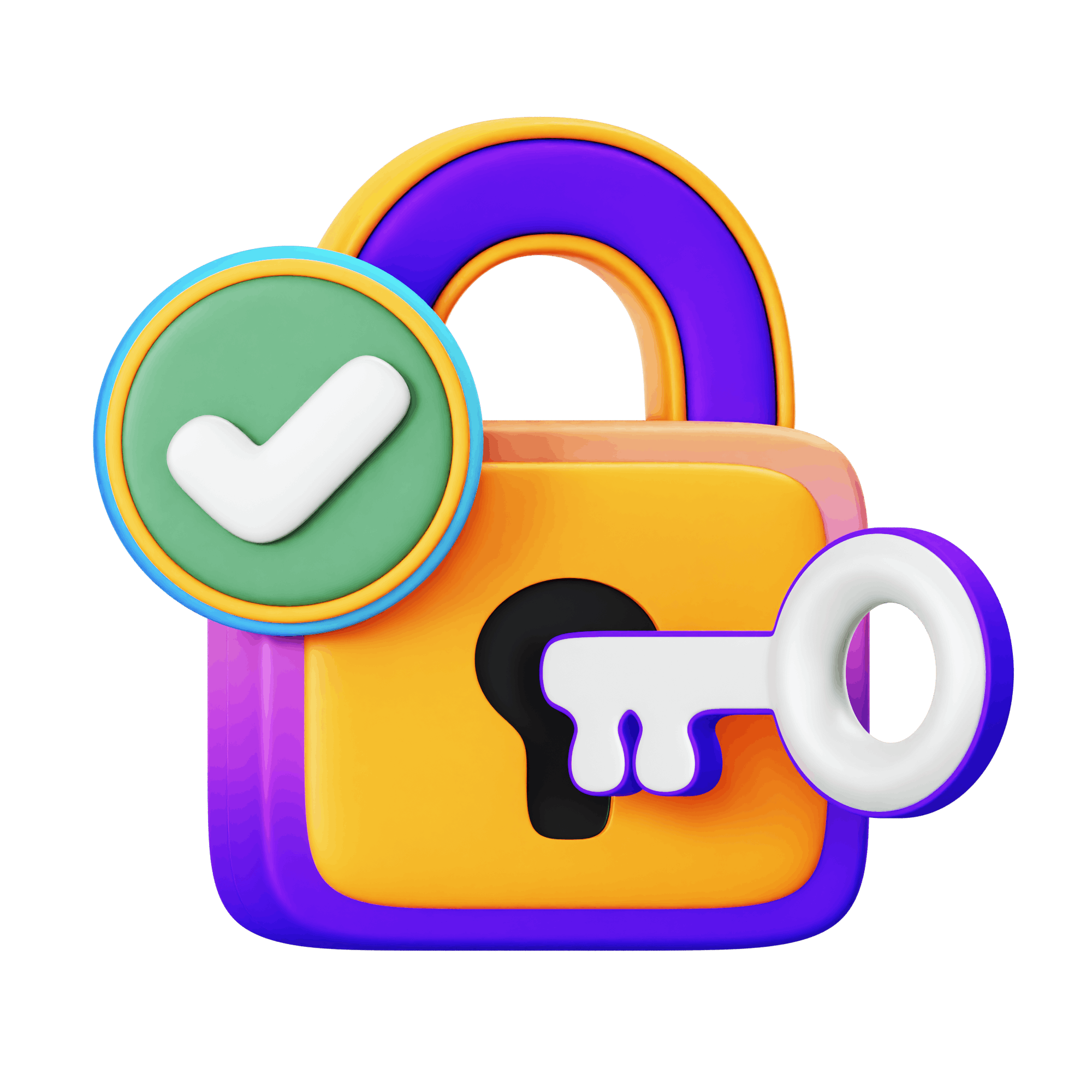
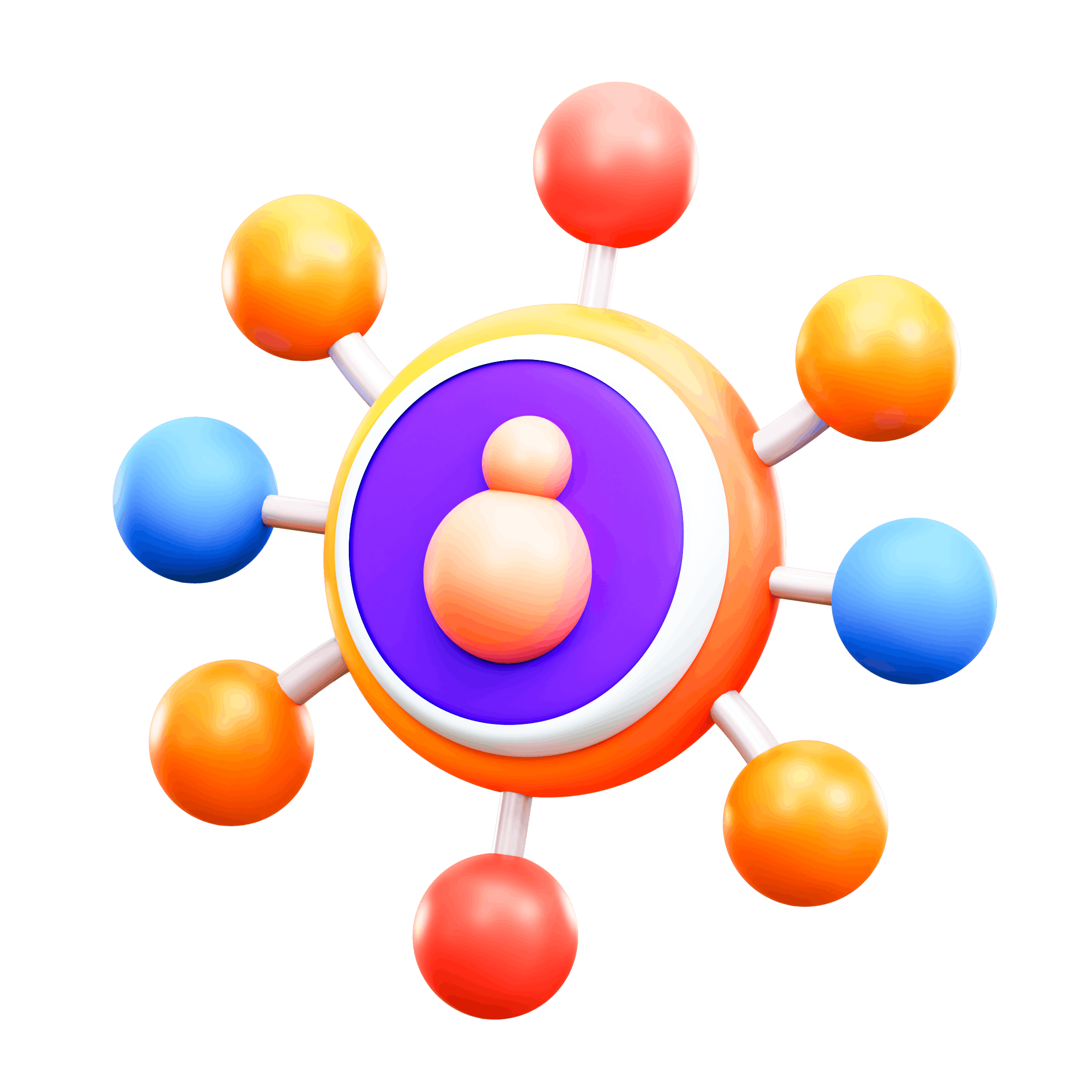
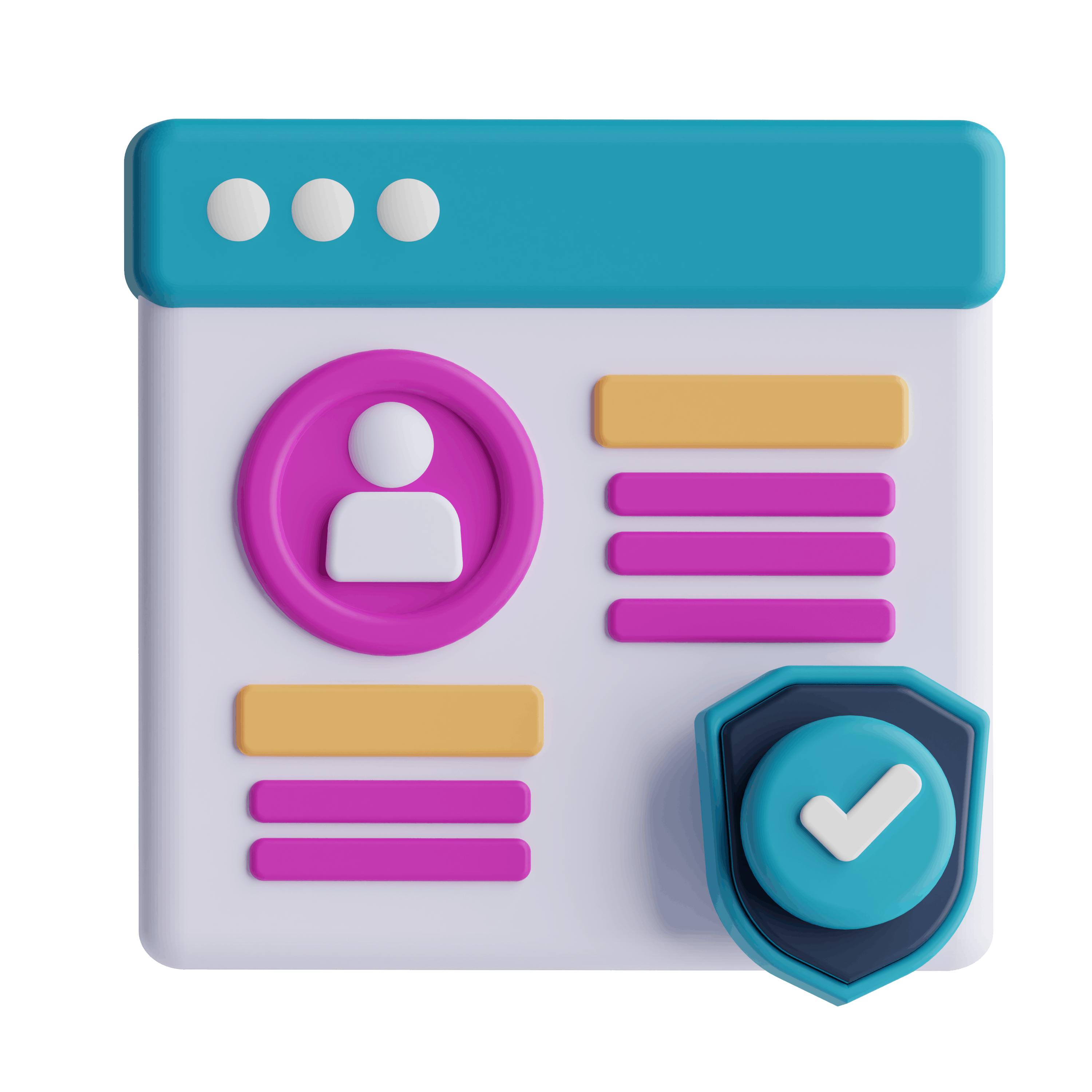
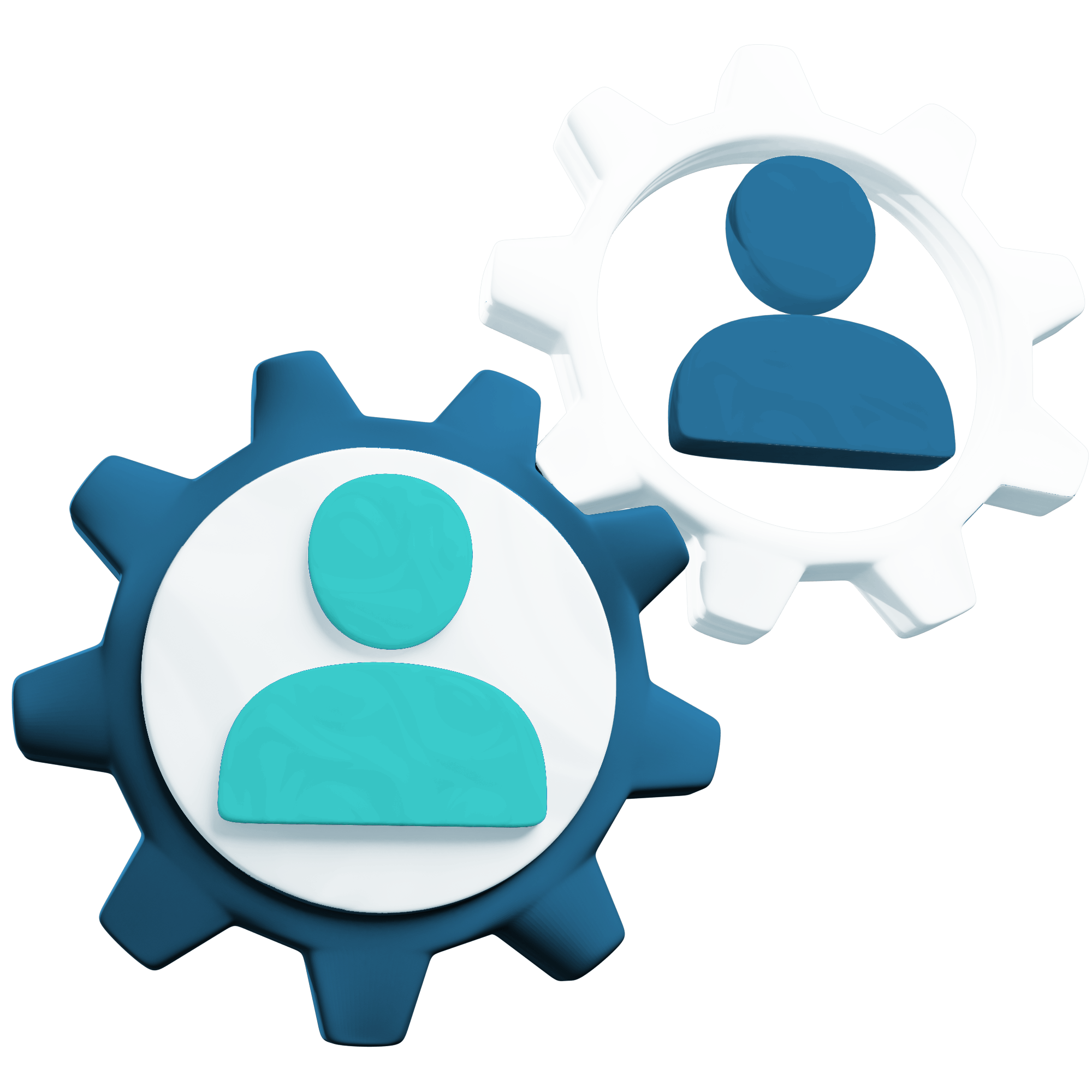
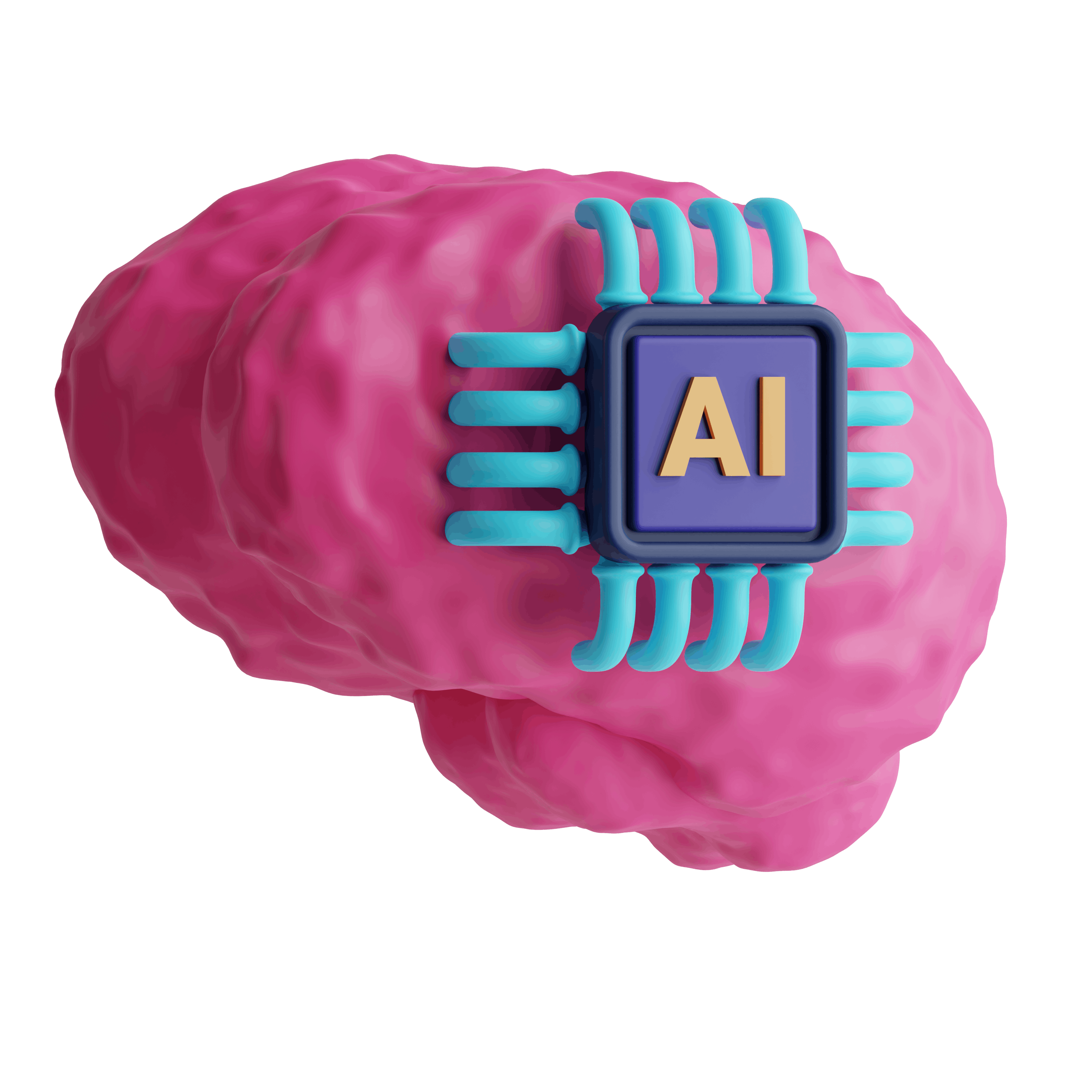
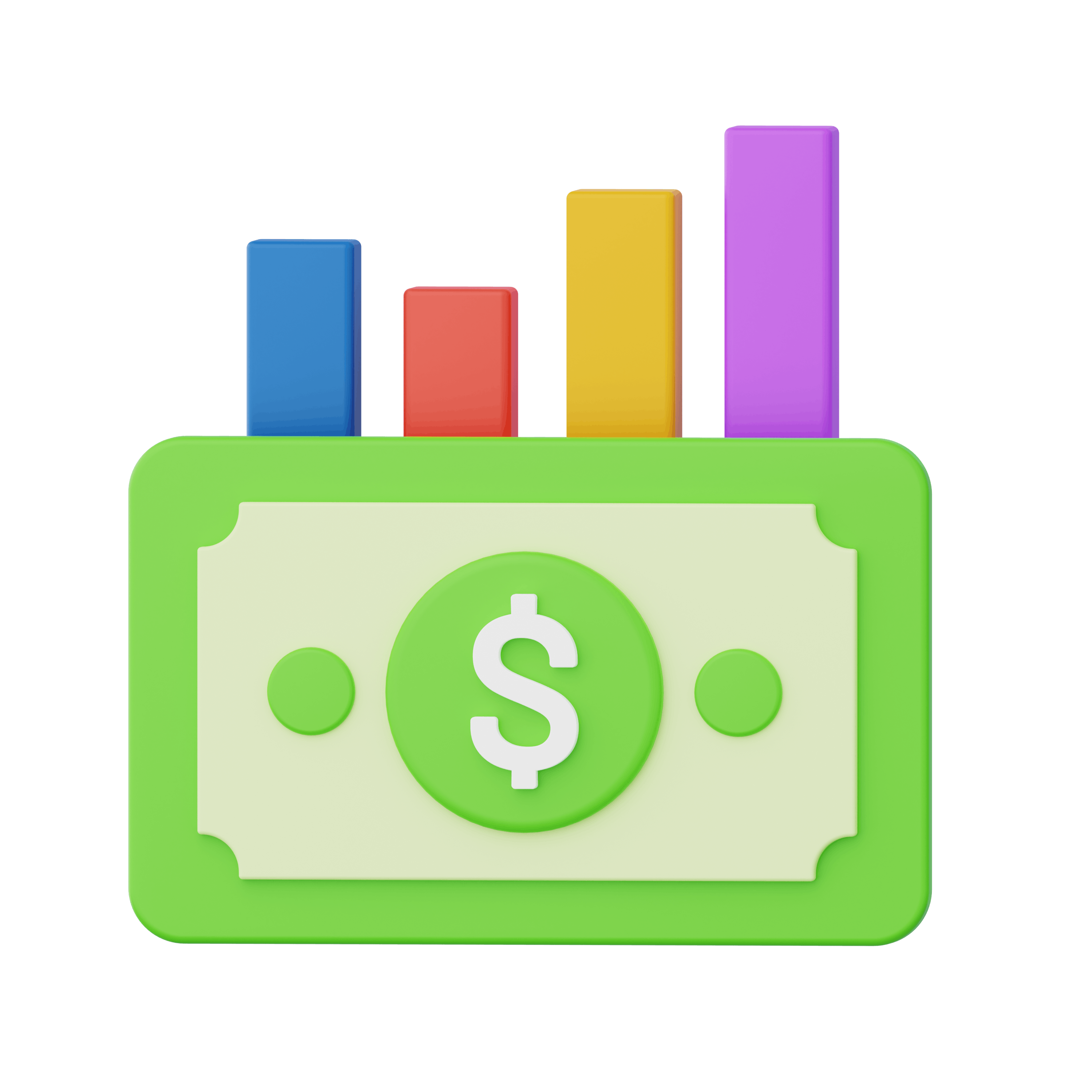
Examples of AI TotalOps : Data, ML & Security
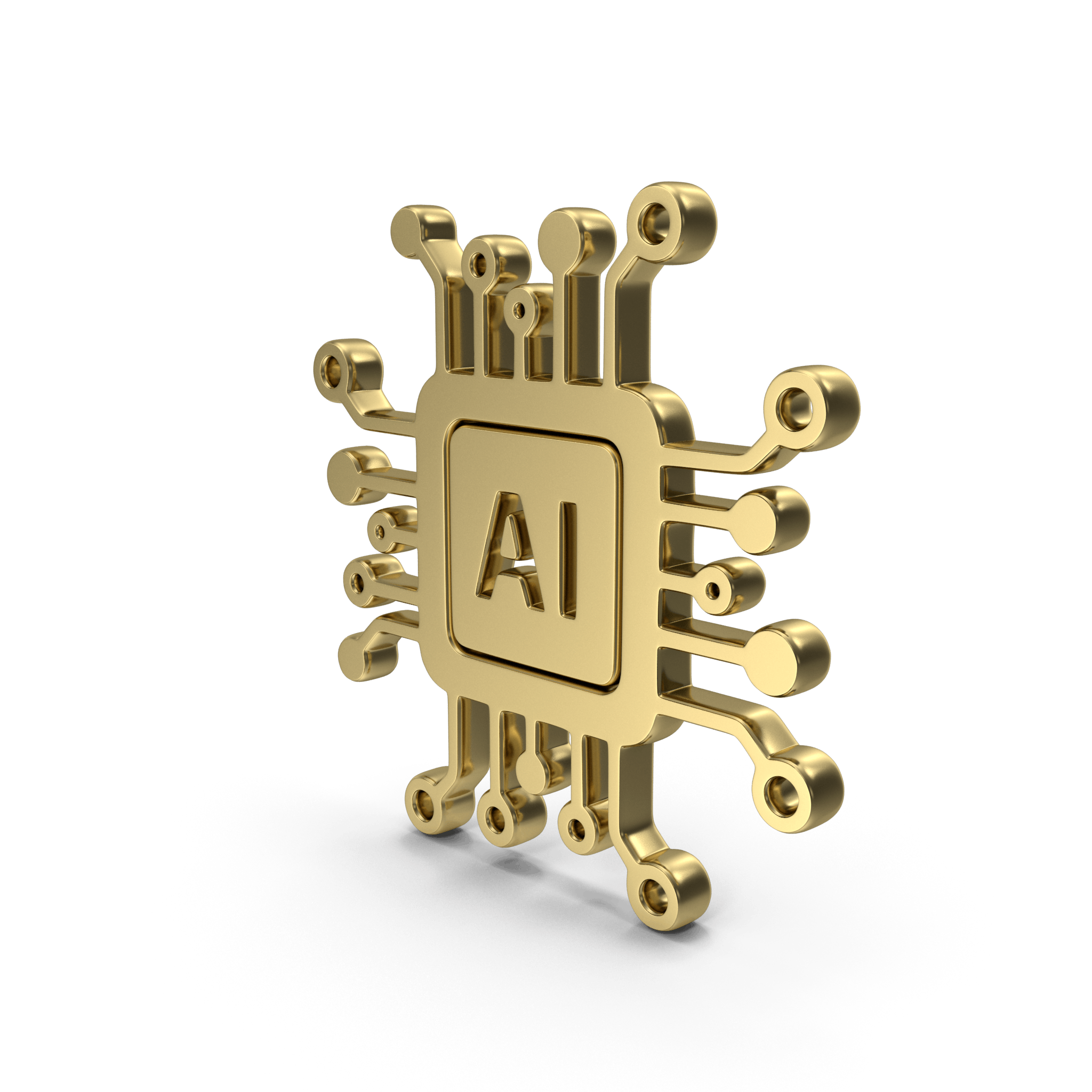

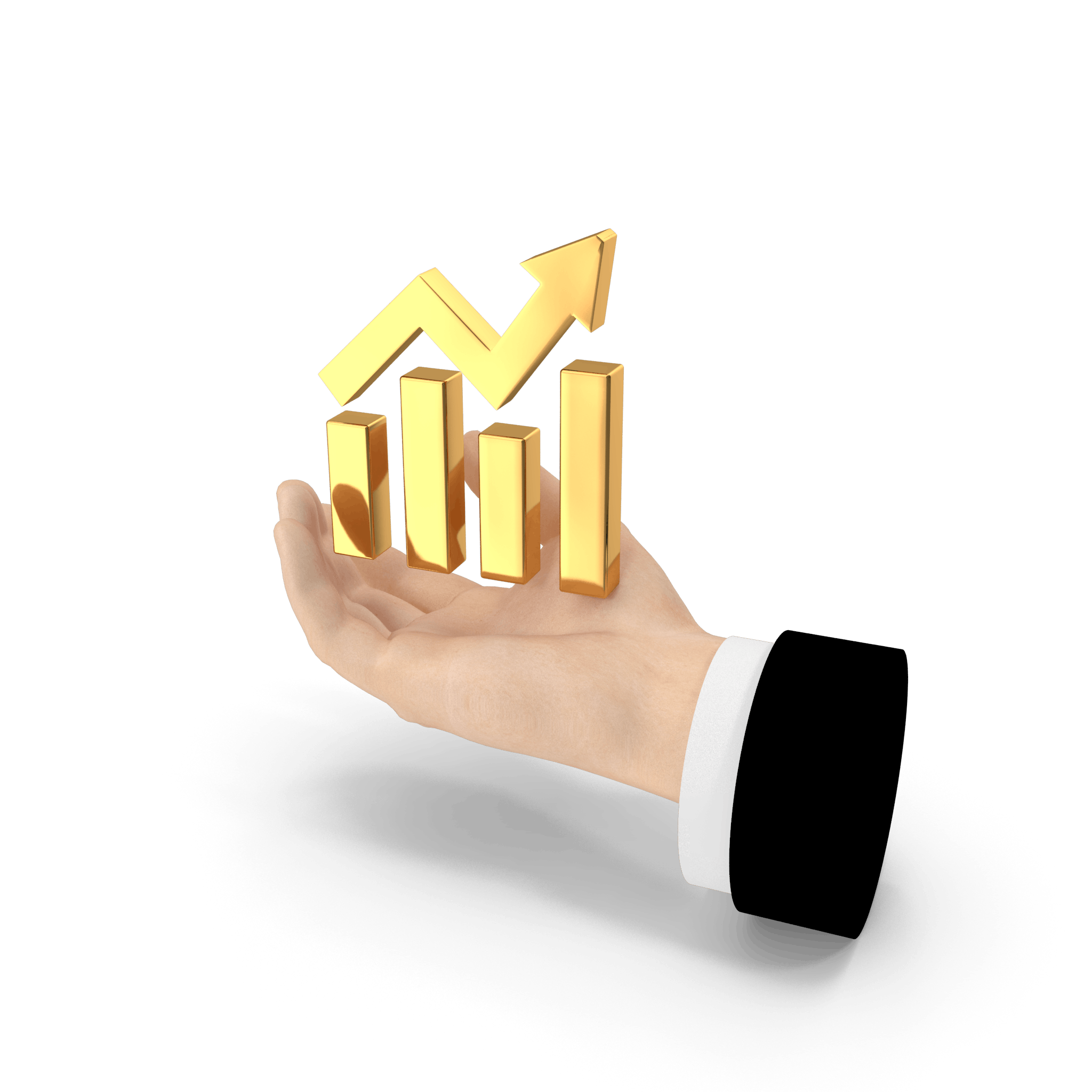
